Biases with AI: A Challenge in Human Resources
AI is revolutionizing HR, but biases in data and algorithms can reinforce inequality. From Amazon’s AI hiring fiasco to LinkedIn’s skewed job ads, real-world examples reveal the risks. Companies must adopt fairness audits, diverse data, and explainable AI to prevent bias. The question remains: Are we using AI to foster equality—or perpetuate old prejudices?
Deepinder Singh
2/18/20253 min read
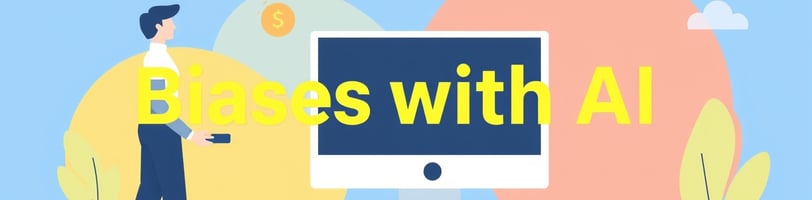
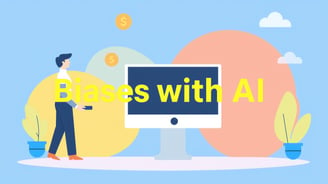
Biases with AI: A Challenge in Human Resources
Artificial Intelligence (AI) is revolutionizing Human Resources (HR) by automating tasks, streamlining recruitment, and improving decision-making processes. However, while AI offers numerous advantages, it also presents a significant challenge: biases. AI-driven HR solutions can unintentionally reinforce and even exacerbate biases if not carefully designed and monitored. Addressing AI bias is essential to ensuring fair, ethical, and effective HR practices.
Understanding Bias in AI for HR
Bias in AI occurs when machine learning models inherit prejudices from their training data, algorithms, or human designers. Since AI systems learn from historical data, they can reflect and perpetuate existing disparities, unintentionally disadvantaging certain groups. In HR, this can manifest in recruitment, promotions, performance evaluations, and workplace culture.
Common Types of AI Bias in HR
Algorithmic Bias – Occurs when the AI model’s underlying logic favors certain groups over others.
Training Data Bias – If historical hiring data is skewed toward a particular demographic, AI models will continue this trend.
Automation Bias – HR professionals may over-rely on AI recommendations without understanding the transparency of the logic.
Selection Bias – AI systems may favor applicants similar to past successful employees, leading to a lack of diversity.
Feedback Loops – If AI models use past hiring decisions as training data, they may reinforce discriminatory patterns.
Real-World Examples of AI Bias in HR
Several high-profile cases highlight the risks of AI bias in HR. For instance, Amazon scrapped its AI-driven hiring tool when it was discovered to be biased against female candidates. The system, trained on resumes submitted over ten years, predominantly from men, systematically downgraded resumes containing words like "women's" (e.g., "women’s chess club"). Read more about Amazon’s AI bias case here.
Another example is LinkedIn’s job recommendation AI, which unintentionally showed high-paying leadership roles more frequently to men than to women. This bias stemmed from historical job application patterns.
How Companies Can Prevent AI Bias in HR
To mitigate AI bias in HR, organizations must adopt proactive strategies, combining technological advancements and ethical guidelines. Below are key steps companies should take:
1. Use Diverse and Representative Data
Ensuring training datasets include diverse demographics helps AI learn fairer decision-making. Companies should periodically audit their data sources to remove any skewed representation.
2. Regular Bias Audits
Frequent algorithm audits help detect and correct biases. Organizations should employ fairness metrics and third-party assessments to ensure neutrality.
3. Explainable AI (XAI)
AI models should be transparent and explainable. Black-box AI decisions can be problematic, as HR professionals must understand how decisions are made to ensure fairness.
4. Human Oversight & AI-Augmented Decision Making
Instead of fully automating hiring, companies should use AI as a decision-support tool. Human HR professionals must critically evaluate AI-generated insights rather than blindly following them.
5. Bias Training for HR Teams
HR professionals should receive training on recognizing and mitigating AI bias. Awareness of how AI models function can help prevent over-reliance on biased recommendations.
6. Collaborate with Ethical AI Experts
Engaging AI ethics consultants or forming AI ethics committees within organizations ensures that AI use aligns with fairness and regulatory standards.
How Technology is Assisting in Reducing AI Bias
Several technological advancements are addressing AI bias in HR:
Fairness-Aware Machine Learning
Developers are implementing fairness-aware algorithms that adjust models to remove discrimination. These models detect and correct imbalances in datasets and predictions.
Bias Detection Tools
New tools, such as IBM’s AI Fairness 360 and Microsoft’s Fairlearn, help organizations assess bias in their AI models and make necessary corrections.
Ethical AI Guidelines and Regulations
Governments and industry bodies are introducing AI ethics guidelines and regulations. The European Union’s AI Act and the U.S. Equal Employment Opportunity Commission’s (EEOC) guidance on AI hiring practices aim to ensure fairness in automated decision-making. Read about the EU AI Act.
Inclusive AI Design
AI developers are increasingly focusing on diverse perspectives when designing AI systems. Inclusive AI development teams reduce the risk of embedding unconscious biases into models.
The Future of AI in HR: A Balanced Approach
AI in HR has enormous potential to enhance efficiency, improve candidate experience, and reduce human bias when used correctly. However, without proper safeguards, AI can reinforce discriminatory practices at an unprecedented scale. The key is to balance automation with human judgment, ethical oversight, and continuous improvements in AI fairness.
As AI continues to shape HR, companies must ask themselves: Are we using AI to break biases or reinforce them? The answer will determine the future of workplace diversity and inclusion.